For a long time, supply chain specialists have been dependent on traditional forecasting models and techniques to estimate demand for their goods and services. Both the digital transformation and the fourth industrial revolution –industry 4.0– are advancing rapidly. This has a fundamental influence on the way human beings live and work. The introduction of digital identifiers and the internet of things (IoT) produced a huge amount of data. One of the main impacts of this situation is that supply chain management decision-making is increasingly driven by data instead of experience. However, the traditional strategies and methods used in the different parts of the supply chain impose many constraints that prevent gaining full advantage of data.
The function demand forecasting plays in supply chain management is related to strategic business planning. Demand forecasting also initiates supply chain management activities such as raw material planning, purchasing, and logistics. It directs the order management and distribution procedures in both push and pull processes.
In the turbulent business world where the competition is fierce, product life cycles get shorter, and consumer behavior changes rapidly, companies require more accurate forecasting methods to surpass the competitors and remain in the market. Traditional demand forecasting tools will never detect a big turning point, an abrupt change in trajectory, or a random shift in momentum. Traditional forecasting is not flexible or fast enough to cope with rapid changes in today's business world. The look to the past years shows that traditional demand forecasting is not enough for today's needs. That is why we are considering a new generation of forecasting models.
Applying AI/ML forecasting in demand planning helps overcome some of these shortcomings and empowers industries with more accurate demand forecasting methods. According to Mckinsey, ML-based forecasting can reduce errors significantly and increase accuracy, leading to a 65% reduction in lost sales and decreasing warehousing costs by up to 40%.
This article tries to shed light on the topic of demand forecasting in the supply chain. It explains the traditional and ML forecasting methods. Moreover, it describes why the application of ML forecasting is inevitable in today's business world.
What is ML forecasting?
Unlike traditional demand forecasting models, a Machine Learning Algorithm takes an input and generates some logic that you can use on fresh inputs to produce forecasts. A software system can learn from data in this scenario to create a better analysis.
One of the major applications of the machine learning model is forecasting. Therefore, you should take specific considerations into account in implementing ML forecasting. The challenges originate from the data's temporal structure because, unlike most ML projects, applying a pre-trained model to fresh data points isn't enough to produce forecasts; instead, as we'll see later in this piece, extra steps are required.
A machine learning methodology, as opposed to traditional demand forecasting methods, allows you to:
- Increase the speed of data processing
- Make a more precise forecast
- Update forecasts automatically based on the latest data
- Analyze more data
- Recognize hidden patterns in data
- Make a strong system with its accuracy
- Increase your capacity to adjust to changes
Machine learning and artificial intelligence are two related technologies that are sometimes used interchangeably; different names sometimes refer to them. The distinctions between ML and AI are listed below.
Artificial intelligence is made from the words "artificial" and "intelligence," which together signify "human-made thinking power." AI is a more general term. Artificial intelligence can learn and train by providing an appropriate set of data.
On the other hand, machine learning focuses on extracting knowledge from the data, making it a more "intelligent" option. It allows a computer system to make predictions or decisions based on past facts without being coded directly. Machine learning uses a large amount of structured and semi-structured data for a machine learning model to produce reliable results or make predictions based on it.
Traditional forecasting and ML forecasting
Traditionally, businesses depended on statistical forecasting approaches like exponential smoothing and linear regressions to help them make decisions. However, machine learning-based forecasting has superseded traditional methodologies in many data and analytics initiatives across businesses and sectors. Machine Learning Forecasting models have been implemented slowly due to storage capacity limitations and lack of proper data. The introduction of cloud computing made the application of ML forecasting more common in different industries.
Machine Learning Forecasting aids Supply Chain and logistics specialists in recognizing and estimating consumer demand more accurately than traditional forecasting techniques.
Challenges in forecasting due to the pandemic
The Covid-19 pandemic's rapid changes in customer demand (For example, empty shelves for some products in supermarkets, changes in consumer demand patterns, etc. )have highlighted the critical need for accurate forecasting and automating forecasting operations within the supply chain. Machine learning technologies have shown to be invaluable in inventory control and demand planning during the pandemic, freeing companies much-needed working capital.
The pandemic proved that traditional forecasting could not provide accurate forecasts to keep inventories low for keeping working capital low.
Impact on different industries
Machine learning systems are implemented slowly in the industry, owing to a lack of expertise among businesses and interaction with standard forecasting software. Despite the difficulties, machine learning is beginning to be used in demand planning in various companies, particularly those with huge inventories to manage. It has impacted various industries, including financial institutions, manufacturing, retail, logistics, legal firms, healthcare, and travel and hospitality.
Need for a more responsive and faster forecast
The impact of Covid-19 on the markets was a rapid and unexpected shock. Businesses could not make solid forecasts in response to the new circumstance due to a lack of data at the outset, regardless of whether they used machine learning or other statistical techniques. Many organizations' demand planning teams resorted to making manual prediction changes based entirely on their market outlook and experience in this challenging situation. While this may have been necessary for the circumstances, at least in the weeks following the first shock, it is time-intensive and frequently wrong.
Forces in the business that drive ML-based forecasting
The forces in the business that drive ML-based forecasting are:
- Trend: Consistent, long-term movement in one direction.
- Seasonality: Seasonality is defined as regular fluctuations with different periods. Most often a year.
- Random Components: When trends and seasonalities are removed from time-series data, and irregular variation emerges.
Machine Learning Forecasting – deep dive
Machine Learning (ML) is a technique for extracting knowledge/patterns from large amounts of data. We can apply machine learning to forecast financial outcomes, predict market supply/demand/inventory, and improve corporate performance. ML can assess historical data to determine demand, supply, and stock and then estimate demand, supply, and inventory in the future.
Machine learning drivers for demand forecasting have advanced to the point where they can produce transformative benefits in the following ways:
- Accuracy, transparency, and depth of analytical options and outcomes are all important factors.
- The ability to consume and exploit a diverse set of data; a system that is "hungry" for data that provides fresh insights
- Processing speed, simple speed/accuracy trade-offs, and workflows that provide data science insights on which data to use and which feature extraction approaches offer improved forecast accuracy
- Overall operational impact on the business, powered by sophisticated algorithms that are simple to configure; when applied in a well-managed environment, this provides robust outcomes.
Recent developments in ML forecasting
- Hyper automation: Almost anything inside a corporation can now be automated, according to the latest trend. All of the company's operations, starting with traditional business processes, should be automated. AI and machine learning fuel hyper-automation.
- Forecasting and analysis of the business: Over the last few years, time series and analysis have become commonplace. With the right strategy, ML can make conjectures at a high rate.
- High-performance computing: High-performance computing accelerates the implementation of ML forecasting in different industries. The way analysts work has altered as a result of artificial intelligence (AI). With pragmatic advancements, the process of algorithmic breakthroughs will continue to accelerate at an astonishing rate. Cloud machine learning (ML) solutions are becoming more popular among businesses.
- Reinforcement learning: Reinforcement learning (RL) is a new term that businesses will widely use in the next few years. It applies deep learning to increase the effectiveness of acquired data by drawing on its own experiences.
Human intelligence refers to a person's academic ability to think, learn from various expressions, comprehend complex ideas, solve numerical problems, adapt to new situations, manipulate one's environment, and communicate with other people. Human intelligence is so remarkable because it is fueled by abstract emotions like energy and inspiration, which enable people to complete complex psychological tasks.
The importance of human decision making
Human intelligence is associated with a wide range of knowledge and experience. It doesn't usually rely on pre-processed data like the ones required for AI. Human intelligence also operates with abstract thinking and logic. Due to this, we can make inferences that are much more advanced than AI can. Human memory and its registering power may appear immaterial compared to the machine's equipment and programming foundation. Humans always have the data much before any machine does. However, our brain's depth and layers are far more unpredictable and complex but often limited.
Artificial intelligence and machine learning have progressed from science fiction to reality, and there is no doubt that artificial intelligence is shaping every industry and propelling the world forward. However, it is still impossible to exactly duplicate human intelligence. Experts believe that computers will not be able to replicate human thought processes in the near future because scientists and experimenters are still baffled by the maze beneath the human thought process. It is not expected that machines will match humans in intelligence in the near future. However, artificial intelligence complements human intelligence.
Challenges in Machine Learning Forecasting
1. Data acquisition: Machine learning requires large data sets to train on, whichmust be comprehensive, unbiased, and high quality. They may also have to waitfor new data to be created at times.
2. Resources and time: ML requires adequate time for the algorithms to learn andmature to the point where they can serve their purpose with a high level ofaccuracy and relevance. It also requires a lot of resources to run. This maynecessitate greater computer processing power on your part.
3. Results interpretation: Another significant problem is appropriately interpreting theresults given by the algorithms. You must also select the algorithms that arebest suited to your needs.
4. Higher chances of errors: Machine Learning is self-contained; however, it is not alwayserror-free. Assume you're training an algorithm with data sets that aren'tlarge enough to be inclusive. As a result of a biassed training set, you end upwith biassed predictions. As a result, buyers are exposed to irrelevantadverts. In the context of machine learning, such errors might start a cascadeof mistakes that go undiscovered for a long time. When they get detected, ittakes a long time to figure out what's causing the problem and even longer tofix it.
A look towards the future of ML-based forecasting
Machine learning isn't just for estimating demand and sales. How skillfully we use this technology in the future will determine its future potential. Today, we focus on demand forecasting technology and realize its added value to modern enterprises by addressing activities such as forecasting customer interaction, future trends, brand development, marketing campaigns, resource utilization, and financial risks. We never know what potential this technology will offer for us tomorrow when new ML trends emerge.
In today's supply chain, AI forecasting is unavoidable, and we should highlight the different reasons for this. For example, obtaining a large amount of data, the need for a quick response to market demand, a shorter PLC, and so forth.
Demand Forecasting with Al/ML assists in the End-to-End (E2E) ecosystem from start to finish, maximizing customer satisfaction, revenue gains, and inventory decreased. Our ML-powered demand forecasting automatically supports all activities on one data platform. Given ML's incremental benefits, companies can use machine learning-powered models to supplement their existing demand forecasting methodologies. It's necessary to move beyond traditional demand forecasting methods and embrace technology.
Machine Learning is extremely powerful when utilized appropriately and in the right places (where large training data sets are accessible), but it is not for everyone.
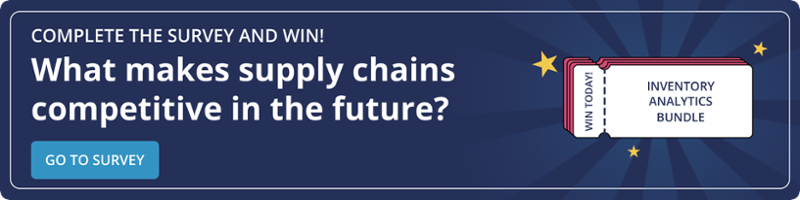